We are pleased to announce the release of our new pipeline for investigating changes in alternative splicing (AS) in RNA-seq. As a quick reminder, a gene may have many forms (isoforms) depending on which exons and transcription start sites are used for transcription. AS is the process by which a cell will preferentially express certain isoforms. Although Basepair offers pipelines for isoform-level quantification, this new pipeline uses LeafCutter, a new, state-of-the-art tool for detecting splicing changes. We always comb through the literature for the best tools, and compared with other AS detection tools, LeafCutter is more accurate and efficient, and it provides intuitive visualizations [1]. Below we highlight some of the key features of our new pipeline:
1. Compare splicing changes between two groups of samples.
The RNA splicing pipeline uses reads mapping to the intron to statistically test for significant differences in novel and known AS events between two sample groups. The alternative splicing events it can detect include: (1) skipped exons, (2) 5′ and 3′ alternative splice site usage, and (3) additional complex events that can be summarized by differences in intron excision / inclusion.
2. Interactively explore your results
The pipeline offers an interactive figure and two tables so you can explore your results. The main table summarizes the per-gene statistical significance of an AS event occurring. Clicking on any row will bring up a more detailed table and visualization. The detailed table will show the exons involved in the AS event, and there will be an interactive figure to visualize those results.
3. LeafCutter as state-of-the-art
Compared with other methods, LeafCutter is focused on intron removal rather than exon inclusion rates, which obviates the need for ambiguous transcript annotations. Compared to other methods, LeafCutter’s approach provides higher accuracy and sensitivity compared to other algorithms. Moreover, it also consumes significantly less time and memory [1].
Validation
Here we demonstrate the validity of the RNA splicing pipeline using a publicly available RNA-seq dataset. The data comes from human brain cells of two groups of patients: (1) a control group consisting of six neurologically normal and five SOD1-ALS (amyotrophic lateral sclerosis) patients and (2) disease group consisting of eight FTD (frontotemporal dementia) patients. This dataset was analyzed before by Conlon et al. [2] and the results are publicly available online.
We used two approaches in our comparison between Basepair results and Conlon et al. However, one caveat for this analysis is we don’t expect our results to exactly match those from Conlon et al. due differences in the alignment pipelines. Nonetheless, the first approach we used was to use the coordinates of the detected AS events to determine the amount of overlap in results (Figure 1). The two sets of results found 68% of the same significant AS events.
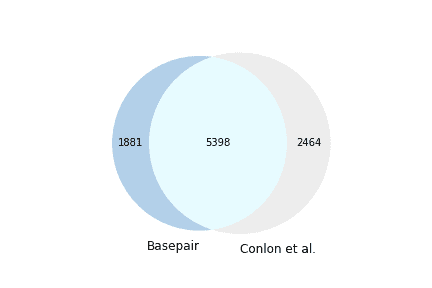
For the second approach, we compared the ΔPSI (delta psi) values for the detected AS events. PSI stands for Percentage Spliced In, which is defined as the average proportion of transcripts that contain a given intron in the sample group. Hence, ΔPSI means the difference in proportion of intron usage between the two groups. Larger ΔPSI values are considered more significant. Figure 2 shows a scatter plot of the ΔPSI values reported by Basepair and Conlon et al. The key results are the strong Pearson correlation (0.972) and R-squared (0.945) between the two sets of results. This indicates Basepair achieved very similar results to those published by Conlon et al.
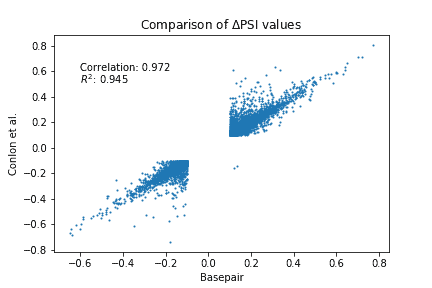
See the pipeline in action
In building any new pipeline, Basepair’s ultimate goal is to help scientists — including those with little to no bioinformatics experience — make sense of genetic data and accelerate their research process. To try out this latest AS pipeline, register for a free account on Basepair. New users can add up to six samples for free and run an unlimited number of analyses on each sample.
References
- Li, Yang I et al. “Annotation-free quantification of RNA splicing using LeafCutter.” Nature genetics vol. 50,1 (2018): 151-158. doi:10.1038/s41588-017-0004-92.
- Conlon, Erin G et al. “Unexpected similarities between C9ORF72 and sporadic forms of ALS/FTD suggest a common disease mechanism.” eLife vol. 7 e37754. 13 Jul. 2018, doi:10.7554/eLife.37754